- Beranda
- penelitian
Literature Review and Theoretical Review of Probabilistic Graphical Models (PGMs)
...
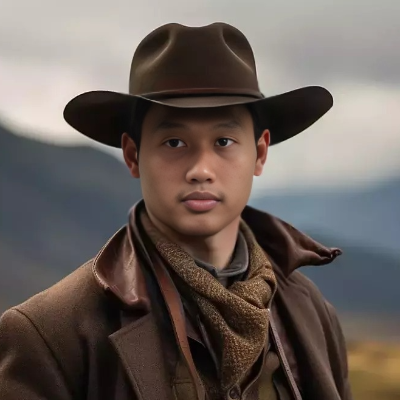
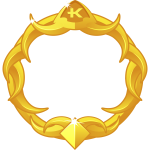
TS
OWNER
yuliuseka
Literature Review and Theoretical Review of Probabilistic Graphical Models (PGMs)
Literature Review and Theoretical Review of Probabilistic Graphical Models (PGMs)
Introduction
Probabilistic Graphical Models (PGMs) are a class of statistical models that represent and reason about uncertainty in complex systems using graphs. These models provide a framework for combining probability theory with graph theory to model and infer relationships between variables in a probabilistic manner.
Literature Review
Historical Development
The field of PGMs has a rich history of development, with significant contributions from various disciplines. Key milestones include:
[color=var(--tw-prose-bold)]Early Foundations (1970s-1980s):
Bayesian Networks (BNs): Introduced by Judea Pearl in the 1980s, BNs represent probabilistic dependencies between variables using directed acyclic graphs (DAGs).
Markov Random Fields (MRFs): Developed in statistical physics, MRFs represent joint probability distributions over random variables using undirected graphs.
Advancements and Applications (1990s-present):
Variational Methods: Development of variational inference techniques for approximate inference in large-scale PGMs.
Learning Algorithms: Introduction of efficient learning algorithms for parameter estimation and structure learning in PGMs.
Deep Probabilistic Models: Integration of deep learning techniques with PGMs to model complex, high-dimensional data.
[/color]
Key Concepts and Techniques
[color=var(--tw-prose-bold)]Bayesian Networks (BNs):
Representation: Directed acyclic graphs where nodes represent random variables and edges denote probabilistic dependencies.
Inference: Exact or approximate inference algorithms such as variable elimination, belief propagation, and Markov Chain Monte Carlo (MCMC).
Applications: Diagnosis, prediction, decision-making, and causal inference in various domains.
Markov Random Fields (MRFs):
Representation: Undirected graphs where nodes represent random variables and edges denote pairwise interactions.
Inference: Exact or approximate inference algorithms such as Gibbs sampling, loopy belief propagation, and graph cuts.
Applications: Image analysis, computer vision, spatial modeling, and social network analysis.
Factor Graphs:
Representation: Bipartite graphs that represent the factorization of joint probability distributions in PGMs.
Inference: Message passing algorithms such as sum-product algorithm and max-product algorithm.
Applications: Turbo codes, decoding algorithms, and sensor networks.
[/color]
Applications of Probabilistic Graphical Models
[color=var(--tw-prose-bold)]Medical Diagnosis: Modeling and predicting disease progression, treatment outcomes, and patient prognosis.
Natural Language Processing: Parsing, semantic analysis, machine translation, and sentiment analysis.
Computer Vision: Object recognition, image segmentation, scene understanding, and image synthesis.
Robotics: Localization, mapping, path planning, and sensor fusion in autonomous systems.
Finance: Risk assessment, portfolio optimization, fraud detection, and market prediction.
[/color]
Theoretical Review
Core Principles
[color=var(--tw-prose-bold)]Probabilistic Modeling:
Bayesian Framework: Formalizes uncertainty using probability distributions and Bayesian inference principles.
Graphical Representation: Represents conditional independence assumptions among variables using graph structures.
Graphical Models:
Directed and Undirected Graphs: Capture causal relationships and pairwise interactions among variables, respectively.
Factorization: Decomposes joint probability distributions into a product of local conditional probabilities or potential functions.
Inference Algorithms:
Exact and Approximate Methods: Exact methods provide the true posterior distribution, while approximate methods offer efficient approximations for large-scale problems.
Message Passing: Propagates beliefs or messages between nodes in the graph to perform inference.
[/color]
Learning Techniques
[color=var(--tw-prose-bold)]Parameter Estimation:
Maximum Likelihood Estimation (MLE): Estimates parameters by maximizing the likelihood of observed data.
Maximum a Posteriori (MAP) Estimation: Incorporates prior knowledge to estimate parameters using Bayesian principles.
Structure Learning:
Score-based Methods: Evaluate candidate graph structures based on goodness-of-fit measures like Bayesian Information Criterion (BIC) or Akaike Information Criterion (AIC).
Constraint-based Methods: Infer graphical structures from data using statistical tests for conditional independence.
[/color]
Evaluation Metrics
[color=var(--tw-prose-bold)]Log-Likelihood: Measures the goodness of fit of the model to the observed data.
Predictive Accuracy: Assesses the model's ability to make accurate predictions on unseen data.
Model Complexity: Quantifies the complexity of the model, balancing model fit and parsimony.
AUC-ROC: Evaluates the performance of probabilistic classifiers in binary classification tasks.
KL Divergence: Measures the discrepancy between the true and estimated probability distributions.
[/color]
Conclusion
Probabilistic Graphical Models provide a versatile framework for modeling uncertainty and dependencies in complex systems. By combining graphical representations with probabilistic reasoning, PGMs offer powerful tools for inference, learning, and decision-making in a wide range of applications.
Keywords
Probabilistic Graphical Models, Bayesian Networks, Markov Random Fields, Factor Graphs, Inference Algorithms, Learning Techniques, Directed Acyclic Graphs, Undirected Graphs, Medical Diagnosis, Natural Language Processing, Computer Vision, Robotics, Finance
Introduction
Probabilistic Graphical Models (PGMs) are a class of statistical models that represent and reason about uncertainty in complex systems using graphs. These models provide a framework for combining probability theory with graph theory to model and infer relationships between variables in a probabilistic manner.
Literature Review
Historical Development
The field of PGMs has a rich history of development, with significant contributions from various disciplines. Key milestones include:
[color=var(--tw-prose-bold)]Early Foundations (1970s-1980s):
Bayesian Networks (BNs): Introduced by Judea Pearl in the 1980s, BNs represent probabilistic dependencies between variables using directed acyclic graphs (DAGs).
Markov Random Fields (MRFs): Developed in statistical physics, MRFs represent joint probability distributions over random variables using undirected graphs.
Advancements and Applications (1990s-present):
Variational Methods: Development of variational inference techniques for approximate inference in large-scale PGMs.
Learning Algorithms: Introduction of efficient learning algorithms for parameter estimation and structure learning in PGMs.
Deep Probabilistic Models: Integration of deep learning techniques with PGMs to model complex, high-dimensional data.
[/color]
Key Concepts and Techniques
[color=var(--tw-prose-bold)]Bayesian Networks (BNs):
Representation: Directed acyclic graphs where nodes represent random variables and edges denote probabilistic dependencies.
Inference: Exact or approximate inference algorithms such as variable elimination, belief propagation, and Markov Chain Monte Carlo (MCMC).
Applications: Diagnosis, prediction, decision-making, and causal inference in various domains.
Markov Random Fields (MRFs):
Representation: Undirected graphs where nodes represent random variables and edges denote pairwise interactions.
Inference: Exact or approximate inference algorithms such as Gibbs sampling, loopy belief propagation, and graph cuts.
Applications: Image analysis, computer vision, spatial modeling, and social network analysis.
Factor Graphs:
Representation: Bipartite graphs that represent the factorization of joint probability distributions in PGMs.
Inference: Message passing algorithms such as sum-product algorithm and max-product algorithm.
Applications: Turbo codes, decoding algorithms, and sensor networks.
[/color]
Applications of Probabilistic Graphical Models
[color=var(--tw-prose-bold)]Medical Diagnosis: Modeling and predicting disease progression, treatment outcomes, and patient prognosis.
Natural Language Processing: Parsing, semantic analysis, machine translation, and sentiment analysis.
Computer Vision: Object recognition, image segmentation, scene understanding, and image synthesis.
Robotics: Localization, mapping, path planning, and sensor fusion in autonomous systems.
Finance: Risk assessment, portfolio optimization, fraud detection, and market prediction.
[/color]
Theoretical Review
Core Principles
[color=var(--tw-prose-bold)]Probabilistic Modeling:
Bayesian Framework: Formalizes uncertainty using probability distributions and Bayesian inference principles.
Graphical Representation: Represents conditional independence assumptions among variables using graph structures.
Graphical Models:
Directed and Undirected Graphs: Capture causal relationships and pairwise interactions among variables, respectively.
Factorization: Decomposes joint probability distributions into a product of local conditional probabilities or potential functions.
Inference Algorithms:
Exact and Approximate Methods: Exact methods provide the true posterior distribution, while approximate methods offer efficient approximations for large-scale problems.
Message Passing: Propagates beliefs or messages between nodes in the graph to perform inference.
[/color]
Learning Techniques
[color=var(--tw-prose-bold)]Parameter Estimation:
Maximum Likelihood Estimation (MLE): Estimates parameters by maximizing the likelihood of observed data.
Maximum a Posteriori (MAP) Estimation: Incorporates prior knowledge to estimate parameters using Bayesian principles.
Structure Learning:
Score-based Methods: Evaluate candidate graph structures based on goodness-of-fit measures like Bayesian Information Criterion (BIC) or Akaike Information Criterion (AIC).
Constraint-based Methods: Infer graphical structures from data using statistical tests for conditional independence.
[/color]
Evaluation Metrics
[color=var(--tw-prose-bold)]Log-Likelihood: Measures the goodness of fit of the model to the observed data.
Predictive Accuracy: Assesses the model's ability to make accurate predictions on unseen data.
Model Complexity: Quantifies the complexity of the model, balancing model fit and parsimony.
AUC-ROC: Evaluates the performance of probabilistic classifiers in binary classification tasks.
KL Divergence: Measures the discrepancy between the true and estimated probability distributions.
[/color]
Conclusion
Probabilistic Graphical Models provide a versatile framework for modeling uncertainty and dependencies in complex systems. By combining graphical representations with probabilistic reasoning, PGMs offer powerful tools for inference, learning, and decision-making in a wide range of applications.
Keywords
Probabilistic Graphical Models, Bayesian Networks, Markov Random Fields, Factor Graphs, Inference Algorithms, Learning Techniques, Directed Acyclic Graphs, Undirected Graphs, Medical Diagnosis, Natural Language Processing, Computer Vision, Robotics, Finance
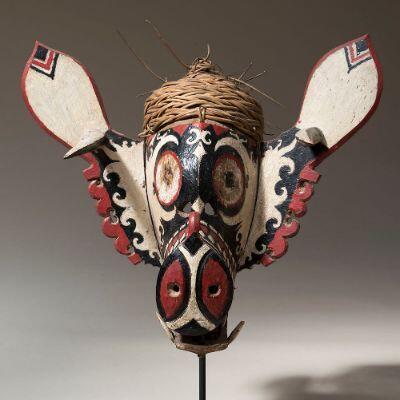
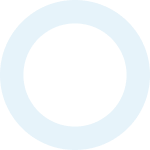
bhintuni memberi reputasi
1
13
2
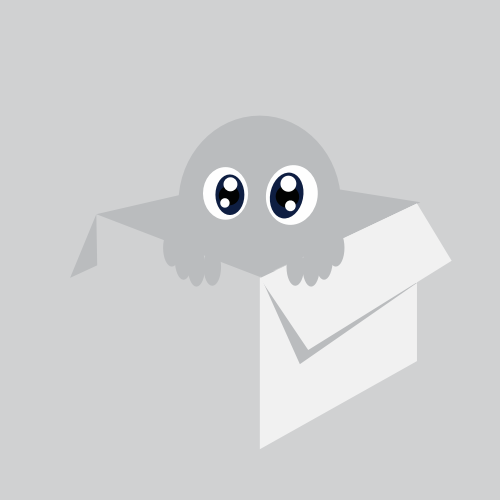
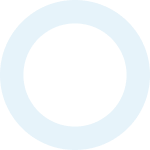
Komentar yang asik ya
Mari bergabung, dapatkan informasi dan teman baru!

penelitian
374Thread•32Anggota
Urutkan
Terlama
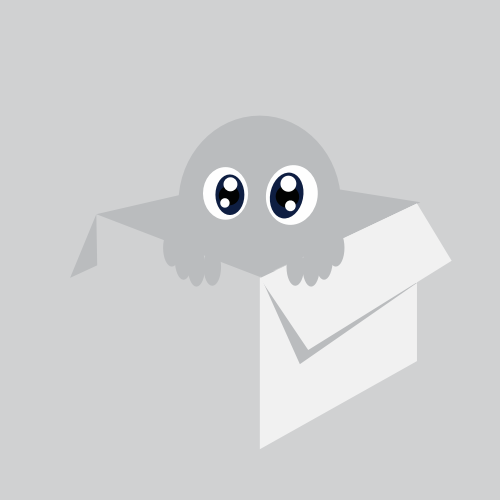
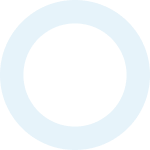
Komentar yang asik ya