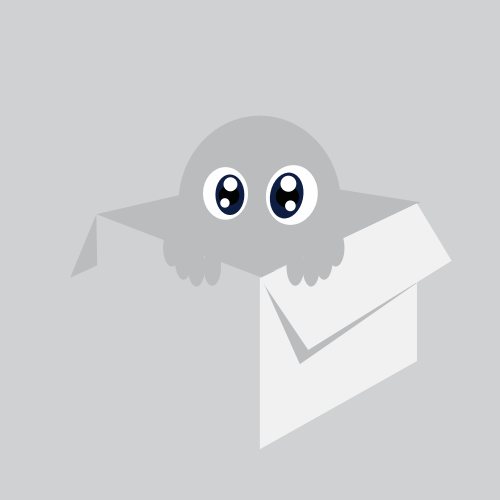
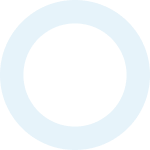
TS
kantharavi51156
How Data Science is Implemented in Finance
From the introduction of bitcoins to the optimization of crucial global economic sectors, finance is one of the fastest-growing businesses. Today, data science makes it possible to conduct financial research and improve financial decision-making. Predictive modeling, clustering, data wrangling, visualization, and dimensionality reduction are some of the most popular approaches used in data science from a scientific perspective. To learn more about the cutting-edge tools, refer to an online data science course in Chennai, co-developed by IBM. Let's examine some of the use of data science in finance in more detail.
How Does Financial Data Science Work?
The broad field of financial analysis uses statistical approaches to understand the problems in finances. The foundations of econometrics are combined with the technical aspects of knowledge science to form financial data science. By leaps and bounds, the Finance desk is expanding.
Prevention of Fraud
Fraud/Scams can occur in various ways, including virtually from your system or digitally, and it indirectly affects numerous businesses. Therefore, it's crucial to deal with these scams and avoid bankruptcy. The main thing they need to do is figure out what caused the scam.
Data analytics, one of the most helpful applications of data science, assists in tracking patterns and surfacing issues considerably faster than humans, enabling the detecting and halting of suspicious behaviors, including speculator trading, rogue trading, and rule violations. The vast datasets and numerous variables processed by machine learning algorithms are used to note hidden correlations between users' behaviors and unrelated behavior.
How can fraud in cybersecurity be detected using data science?
The answer is very straightforward since fraudsters, despite using a variety of tools and techniques, leave a trail of transactional and behavioral data that helps detect fraud. While using Data Analytics to gather data and build predictive models, it is still very simple to handle the volume of data. Data science allows us to gather information from records, such as emails, social media posts, contact center notes, or agent reports. It also aids in identifying anonymous scams and tracking down altering tendencies.
Data Analytics for Taxation Fraud Detection
Many people find that filling out duty returns is stressful. Some people worry about committing serious offenses, while others worry about filing false tax returns. Both might motivate individuals to visit a doctor. It is crystal clear that fraudulent refunds put more of a strain on both the government and honest taxpayers.
Managing Risk
Simulated exposures to and values of credit and marketing risks are frequently more accurate, assisting banks and financial companies in proactive risk monitoring across organizational mainframes.
Due to the widespread exposure of a flaw in conventional risk management techniques in 2008, there has been an increase in financial regulation and a reduction in risk variables. Although data science aids businesses in finding creative ways to live and manage risk across the enterprise,
Financial organizations like banks rely on data science to identify and address fraud. For future benefit, copies of all correspondence between the bank and the customer are kept. So if a serious incident occurs, it will be simpler to spot the fraud and put a stop to it before it causes significant harm to the reputation of the brand or individual.
Customer Analytics
Customer experiences and personalization are now top priorities for almost 30% of financial organizations. With knowledge of science and real-time analytics, they are prepared to glean insights into customer desires as they identify histories and hierarchies.
Additionally, to develop a better business strategy or provide advice to customers that support their preferences for banking or investing.
Similarly, insurance businesses utilize supervised ML to comprehend the factors influencing consumer behavior, cut losses by removing clients with below-zero value, increase cross-sale prospects, and proportionately allocate customers' total lifetime value. Moreover, clustering techniques simplify identifying client groups that exhibit similar behaviors.
Algorithmic Trading
In essence, algorithmic trading is all about intricate mathematical formulas and quick calculations helping businesses create new trading techniques. Underlying data streams can be measured using data science. Massive datasets are incorporated and processed by an analytical engine in the backend to check market predictions continuously.
The following information is used in the form of data by algorithmic trading:
Algorithmic trading refers to using computer programs and analyses that initiate and exit transactions per prescribed parameters such as price changes or volatility levels. Trading algorithms can execute buy or sell orders on your behalf once the current market conditions diverge from any established criterion, enabling you to profit easily from safe and foreseen investments.
If you are interested in becoming a data scientist or analyst in the financial sector, check out the instructor-led data science training in Chennai, offered by Learnbay.
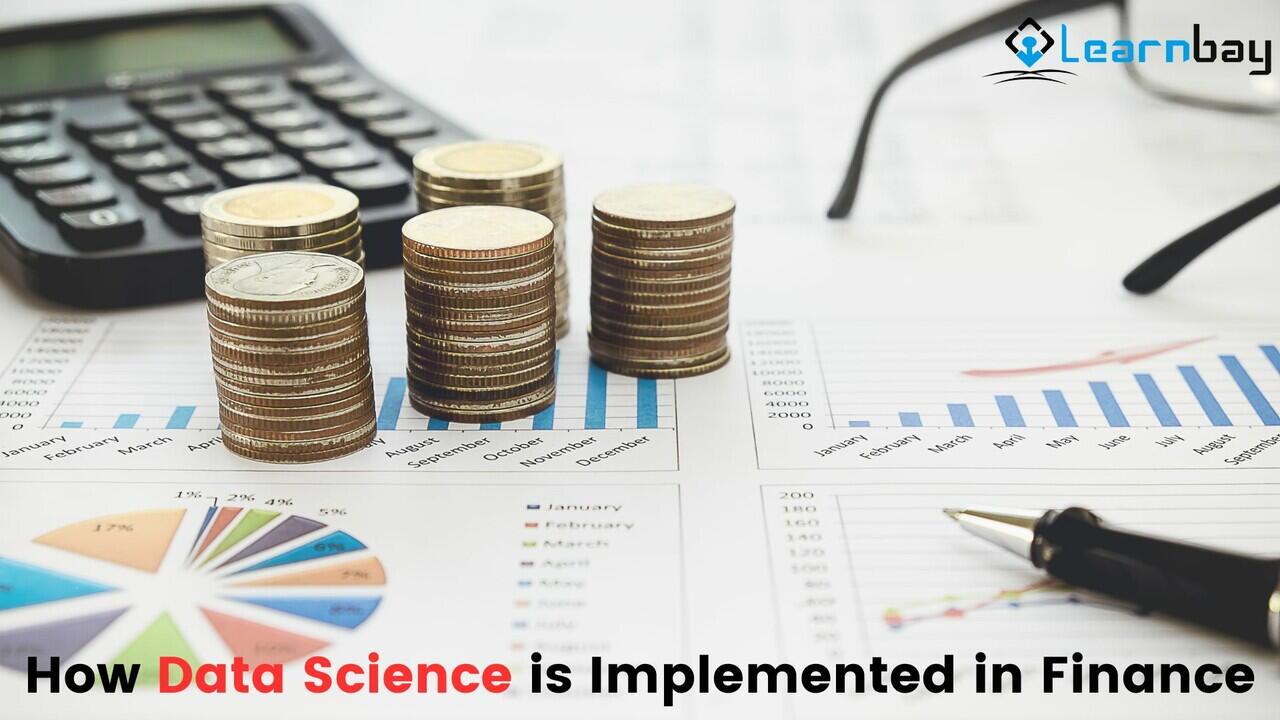
How Does Financial Data Science Work?
The broad field of financial analysis uses statistical approaches to understand the problems in finances. The foundations of econometrics are combined with the technical aspects of knowledge science to form financial data science. By leaps and bounds, the Finance desk is expanding.
Prevention of Fraud
Fraud/Scams can occur in various ways, including virtually from your system or digitally, and it indirectly affects numerous businesses. Therefore, it's crucial to deal with these scams and avoid bankruptcy. The main thing they need to do is figure out what caused the scam.
Data analytics, one of the most helpful applications of data science, assists in tracking patterns and surfacing issues considerably faster than humans, enabling the detecting and halting of suspicious behaviors, including speculator trading, rogue trading, and rule violations. The vast datasets and numerous variables processed by machine learning algorithms are used to note hidden correlations between users' behaviors and unrelated behavior.
How can fraud in cybersecurity be detected using data science?
The answer is very straightforward since fraudsters, despite using a variety of tools and techniques, leave a trail of transactional and behavioral data that helps detect fraud. While using Data Analytics to gather data and build predictive models, it is still very simple to handle the volume of data. Data science allows us to gather information from records, such as emails, social media posts, contact center notes, or agent reports. It also aids in identifying anonymous scams and tracking down altering tendencies.
Data Analytics for Taxation Fraud Detection
Many people find that filling out duty returns is stressful. Some people worry about committing serious offenses, while others worry about filing false tax returns. Both might motivate individuals to visit a doctor. It is crystal clear that fraudulent refunds put more of a strain on both the government and honest taxpayers.
Managing Risk
Simulated exposures to and values of credit and marketing risks are frequently more accurate, assisting banks and financial companies in proactive risk monitoring across organizational mainframes.
Due to the widespread exposure of a flaw in conventional risk management techniques in 2008, there has been an increase in financial regulation and a reduction in risk variables. Although data science aids businesses in finding creative ways to live and manage risk across the enterprise,
Financial organizations like banks rely on data science to identify and address fraud. For future benefit, copies of all correspondence between the bank and the customer are kept. So if a serious incident occurs, it will be simpler to spot the fraud and put a stop to it before it causes significant harm to the reputation of the brand or individual.
Customer Analytics
Customer experiences and personalization are now top priorities for almost 30% of financial organizations. With knowledge of science and real-time analytics, they are prepared to glean insights into customer desires as they identify histories and hierarchies.
Additionally, to develop a better business strategy or provide advice to customers that support their preferences for banking or investing.
Similarly, insurance businesses utilize supervised ML to comprehend the factors influencing consumer behavior, cut losses by removing clients with below-zero value, increase cross-sale prospects, and proportionately allocate customers' total lifetime value. Moreover, clustering techniques simplify identifying client groups that exhibit similar behaviors.
Algorithmic Trading
In essence, algorithmic trading is all about intricate mathematical formulas and quick calculations helping businesses create new trading techniques. Underlying data streams can be measured using data science. Massive datasets are incorporated and processed by an analytical engine in the backend to check market predictions continuously.
The following information is used in the form of data by algorithmic trading:
Algorithmic trading refers to using computer programs and analyses that initiate and exit transactions per prescribed parameters such as price changes or volatility levels. Trading algorithms can execute buy or sell orders on your behalf once the current market conditions diverge from any established criterion, enabling you to profit easily from safe and foreseen investments.
If you are interested in becoming a data scientist or analyst in the financial sector, check out the instructor-led data science training in Chennai, offered by Learnbay.
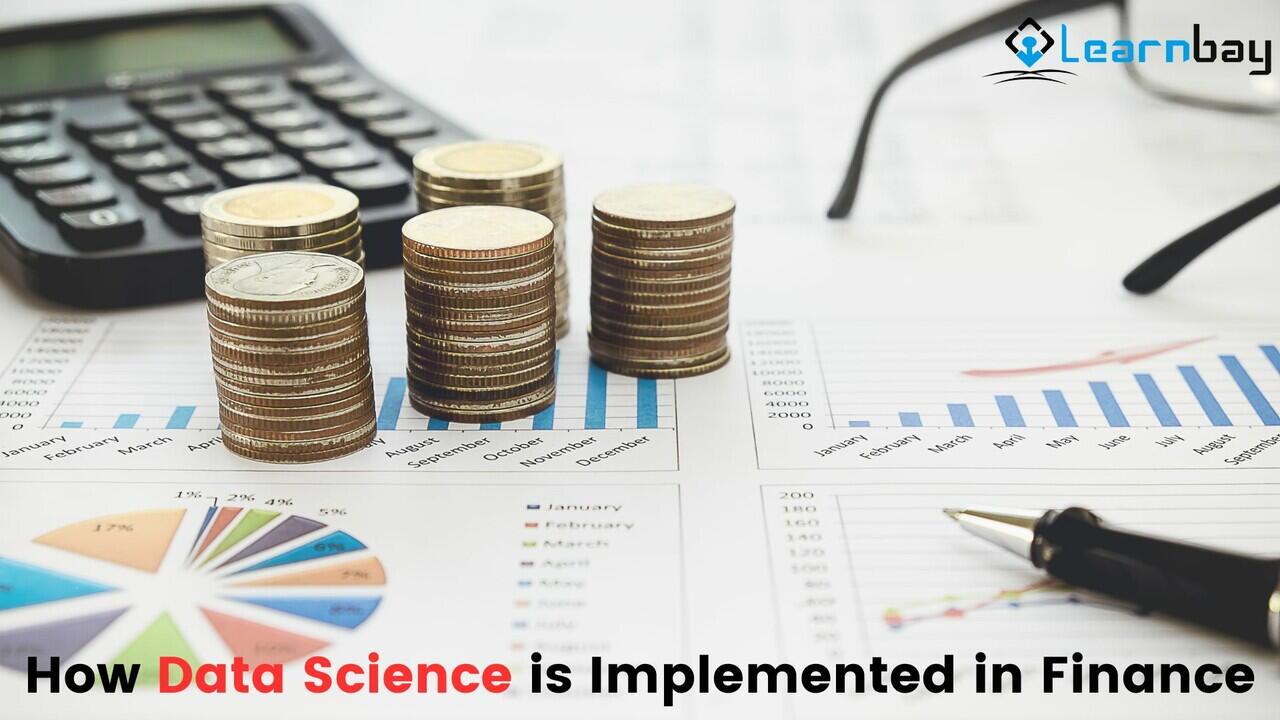
Data science course in chennai
0
99
0
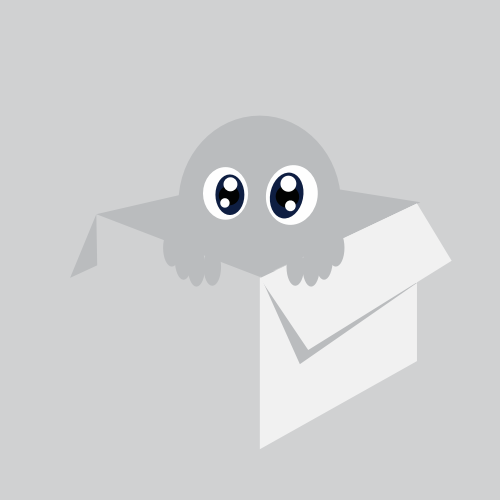
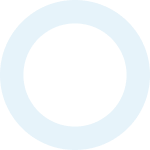
Komentar yang asik ya
Mari bergabung, dapatkan informasi dan teman baru!

Education
22.6KThread•13.7KAnggota
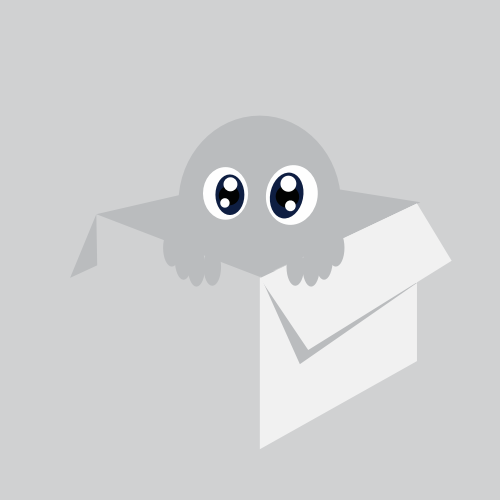
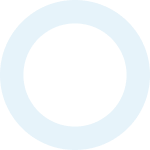
Komentar yang asik ya